Last modified on February 20, 2024
CLUSTER 5: KEY ENABLING TECHNOLOGIES
Introduction
Cluster 5 focuses on CCAM R&I actions on enabling technologies, driven by digitalisation and extending the application of these technologies beyond the individual vehicle in a system’s approach. In case of e.g. AI, it has the capacity to go way beyond on board decision making, based data from in-vehicle sensors. Data from other vehicles, infrastructure and back offices can be used in decision making in complex scenarios including safety critical situations, ranging up to traffic management, emission management, charging of vehicles and provision of many new and emerging mobility services. Thus, the Cluster fosters cooperation amongst stakeholders from several technology areas and industries. Safe and secure operation of vehicles and mobility systems is key to develop trust and establish user adoption of CCAM solutions.
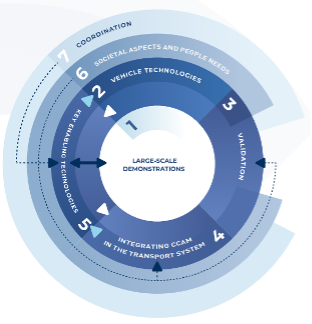
Cluster 5 Objectives
- Provide AI and cybersecurity solutions to be integrated in vehicle technologies (related to environment perception and on-board decision making) (to Cluster 2).
- Develop harmonised procedures, methods and tools for the validation of cyber security for inclusion in conceptual description of an approval scheme of CCAM systems (to Cluster 3).
- Provide the basis for validation methods and tools for AI applications in CCAM for integration in validation procedures for CCAM systems (to Cluster 3).
- Develop and/or integrate Key enabling technologies (e.g. secure communication, robust and resilient connectivity, edge computing) contributing to better performance of vehicle-transport system integration (to Cluster 4) and of in-vehicle technologies integration in the cloud-edge continuum.
- Elevate the possibilities for synergies with stakeholders, also beyond originally mobility focused partners, using enabling technologies to take a systems’ perspective in road mobility and CCAM Technologies.
A harmonised approach to further develop these technologies can help to reduce market fragmentation, currently hindering EU companies to fully benefit and exploit new mobility business cases based on CCAM. For this, the extended domains are addressed with R&I actions for cybersecurity, information and data sharing and artificial intelligence (AI). Advancing CCAM solutions and developing a common understanding of well-functioning CCAM under all circumstances will certainly benefit from harmonising approaches for data sharing (aligned with the EU Data Act), whilst on the other hand leading to maximised societal benefits of the technology application. Harmonised approaches for data sharing, leading to a system architecture for data sharing, should be developed for the full range of product development stages from development, testing, and validation up to implementation. With these developments, it is important to differentiate data from information, in which the latter is a dataset collectively carrying a logical meaning. As data and information sharing is advancing, it is essential to include data security and cybersecurity requirements complying with European regulation (The Data Governance Regulation) regarding privacy, data security and cybersecurity. Fragmentation of data sharing approaches would lead to lack of seamless mobility possibilities, duplication of data storage and huge data inefficiencies, lack of coherence.
Extending the system domains beyond the vehicle through connectivity (short range or cellular) makes cybersecurity a fundamental building block for trusted (digital) interaction of road users with each other, the infrastructure and cloud-based solutions/services. CCAM technologies will more and more use data from multiple sources, and will need the capabilities to integrate this data. Cybersecurity needs to be made an integral part of the development process, with common aims and objectives, frameworks/architectures, and designs (including normal operation, decision making and actuation as well as anomaly detection). Furthermore, it should lead to the advancement and development of new solutions for assessing resilience at distributed and at a system level. These techniques are needed to enhance vulnerability detection and robustness of CCAM system. The target will be to develop tools and methods to detect and mitigate all possible attacks and their cascading effects in the CCAM system. Improved AI-based cybersecurity attack detection to in-vehicle networks, with more precise identification and attack attribution, and potential response will require major actions, needed to ensure safe and secure operation. Connectivity will to an increasing extent use new solutions, like 6G when it’s deployed wider; joint actions with the SNS Partnership can help to take a coherent approach here.
AI has a huge potential to change the (in-vehicle) decision making process as well as new services or safety solutions supported by AI, especially when a systems’ approach is taken. AI has to become a functional competitive and affordable CCAM technology first, in order to support safe autonomous driving in a more complex and dense traffic environments. Specific automotive requirements such as safety, security and real-time functionality demand dedicated AI development for CCAM applications. This will have to go hand in hand with human centric AI developments, delivering guidance on Explainable AI, notions of fairness, and on AI and human interaction, ethics and legal issues. Implementing AI for situational awareness, in particular in CCAM, presents a variety of challenges, e.g. industrialisation, requirement-based development, continuous improvement of trained modules for application in safety critical domains. Verification of AI for situational awareness is essential for CCAM to operate in more complex urban traffic scenarios. The ambition is to move from reactive and/or adaptive system support to AI based predictive system state awareness, decision making and actuation. AI based joint learning approaches for CCAM technologies can bring accelerated improvements.
Digitalisation is an ongoing development in almost all European sectors, also having a clear impact on the CCAM developments, testing, validation, implementation and operations. As such, the effects of digitalisation go beyond the well-established digitalisation in e.g., development processes in automotive. This is reflected in the developments regarding the software defined vehicle (SDV), in which software to an increasing extend is shaping the vehicle customer experience, offering adjustments to the driver’s needs and a potential for constant optimisation of the vehicle.
To make use of the benefits the advancing levels of digitalisation can offer for road mobility, the sector faces major challenges in adopting Artificial Intelligence (AI), Big Data (BD), communication technologies and availability, cybersecurity, and Edge/Cloud Computing. It requires the adoption of models that parallelise and iterate development and operations (DevOps) on a continuous basis, particularly applied to hybrid cloud/backend and edge infrastructures involving Machine Learning processes (MLOps). Consequently, there is a clear need for efficient and cost-effective smart distributed and semantically aware processing tools and a flexible software infrastructure allowing distributed processing (edge/cloud) for data discovery, collection and processing alongside the product lifecycle, which match the constantly growing amounts and varieties of data.
Data plays a crucial role in the AI development and testing. Automated driving requires billions of test kilometres and huge amounts of data, generated through real and simulated test drives. Instrumented vehicles, equipped with cameras, lidar, radar, GNSS/IMU, etc. and with ADAS sensors, gather raw information. The processing of this data to create information will permit its use in the AI pipeline. However, AI-related data management is a complex challenge, mainly due to the heterogeneity of data types, integration of real and synthetic data, coexistence with iterative AI development and operation cycles, and storage and computational costs. Furthermore, unknown sets of non-acquired data can widely influence the correlations created by AI and ML processes, thus producing negative effects in final data driven systems. Data sharing approaches so far are merely limited to project or pilot base, or limited to specific use or Use Cases. For implementing connected, cooperative and automated mobility on a larger scale, including the essential further R&I activities, data sharing (as a key element of theDevOps approach) needs to be established on a wider scale and in an harmonised approach. Serving as a basis for next steps in R&I activities are the best practice examples for test data sharing as they have been developed and reported in the projects L3Pilot, Hi-Drive, ARCADE, SUNRISE and FAME, as well as 5G-Mobix. Inputs from Cluster 7 are needed here. Cross project sharing will be an important first step; initially of data sharing frameworks and architectures, later on also on the actual data sharing and storage. An explicit cooperation will exist with activities within Cluster 1 on large-scale testing as well as with Cluster 4 especially on Connectivity and Cooperative Systems. The activities here should be seen in the light of e.g. Common European Data Spaces and the expected Data Act and take this to the contextual application. Cyber security is often discussed and researched in a general approach. Explicit translation and implementation of results towards the specific CCAM domain needs to be achieved. Research can build upon previous work e.g. the CEN-CENELEC Cyber Security Focus Group. For CCAM, contextual definition is needed, including sector specific security features. This can build further on the recently developed UNECE WP.29 R155 and R156 regulations and ISO/SAE 21434 standard on a structured process to ensure that cyber security is included upfront in the system (vehicle or infrastructure) design. The SECREDAS ECSEL project offers a referential starting point for more sector specific work, while looking at the operational security. The SELFY Project is developing a toolbox made of collaborative tools with the aim of increasing the security, protection and resilience of the CCAM ecosystem against cyber-attacks. Links are included also to Cluster 3 on e.g. v2x communications and validation of over the air updates and programming of vehicles, in a cyber secure approach. Alignment and cooperation is needed with Cluster 2, especially Safe and reliable on-board decision-making technologies, as well as for issues like over air updates of in-vehicle technologies.
CCAM solutions have benefited from the applicability of AI-based perception, situational awareness (outside traffic and inside driver state), and decision-making components. Current state of the art within AI has a primary focus on vehicle state. However, first steps have been made to incorporate information and predictions also regarding human (driver) state (AWARE2ALL project) and system state (the projects EVENTS and ROADVIEW) regarding the environment around the vehicle, including object classification in perception technologies.
The AITHENA and AI4CCAM projects are advancing towards trustworthy AI exploring trade-offs amongother equally important properties. CONNECT project, working on creating fundamental building blocks of a trust modelling framework for CCAM, adds other essential parts to the tool sets available.
Adapted testing mechanisms for trustworthy-AI need to be defined for homologation procedures. These mechanisms shall be compliant with the Data Act and AI Act and firmly address the recommendationsof the AI High Level Expert Group and their Guidelines for Trustworthy AI [3]. In addressing this, close interaction between Cluster 5, Cluster 2, Cluster 3 and Cluster 6 will be needed. Overarching developments like the creating of the software defined vehicle are building on these steps and inputs.
[3] Ethics guidelines for trustworthy AI, 8.04.2019, https://ec.europa.eu/digital-single-market/en/news/ethics-guidelines-trustworthy-ai
AI development and evolution consists of two main steps: training, (teach the algorithm with labelled data), and application (apply the learnt mechanism to newly unseen data). New advances in training like Federated Leaning should be further explored to optimized centralized cloud base training with a smart distribution of the training task across several computing nodes, each of them managing local data, and merging the multiple trained models. Thus, federated learning can facilitate compliance with the principle of data minimization. Inference phase will depend on the (edge) target HW. The implementation of real time self-contained AI functions in the edge (vehicle, infrastructure) is paramount in CCAM specially for critical safety related functions where driving context and decision making needs to be performed without the latency of a cloud transmission. Advances in Edge AI model optimisation, minimizing model energy consumption is required.
The Partnership aims to go beyond static AI systems by moving to adaptive system support and up to AI based predictive system state awareness, decision-making and actuation. Data fusion needs enrichment with also high precision localisation, end-user information, infrastructure (sensor) data as well as system state information, while balancing interactions. Major steps can be made in the broad implementation of CCAM solutions with AI components, for passenger vehicles, logistics providers, traffic management systems and new mobility service offerings, taking an explicit multimodal systems approach. The work within this context can build partially on projects within the framework of the Partnership AI, Data and Robotics and the EC funded project AI4EU. Within the CCAM Partnership, close interactions are foreseen with Cluster 2 especially on environment perception technologies for CCAM, and safe and reliable on-board decision-making technologies.
To enable remote management, real time transmission and display of high quality video, sensor data and commands are required. This imposes new challenges to communication technologies where the latency, bandwidth and communication channel reliability will be of paramount importance Communication link needs to be available and predictable (availability and availability conditions) and should be part of the ODD definition. As such, IoT technologies play a significant role in the implementation of CCAM services and applications. IoT can provide and enrich CCAM services with the integration of road infrastructure information and with other information that can have an impact on the CCAM services (weather conditions, accidents, traffic flows, crowded areas, proximity of vulnerable road users…). Thus, there is a need for research on IoT technologies for communication, sensing and, in particular, precise positioning and tracking, and on intelligent sensors and actuators that can help to generate better contextual information for the development of future CCAM services and applications.
Cluster 5 R&I Actions
The specific R&I actions relating to “Key Enabling Technologies” are the following:
- Cybersecure components and systems. Systems for CCAM enabled mobility will have to be fail-operational and cyber-secure in their entire Operational Design Domains (ODD), to guarantee a safe and secure operation of vehicles and systems. Both safety and cyber-security need to be considered in a systems perspective, also as they are related to the interaction of vehicles as well as with the infrastructure, transport management systems and service systems. Joint definitions of the targeted levels of safety and security will be needed, as well as harmonised methods and protocols for its validation, to be included in e.g., future type approval schemes, for these systems as separate technical units.
- Robustness and resilience. Highly automated vehicles will need consolidated robustness and security measures. Threats, failures, problems as well as malicious and unintended interactions will have to be detected real-time and fed to a decision-making process on response actions. A tool suite of appropriate actions is needed to enable the component or system stepping back in real-time to a safe and secure situation. Improved AI-based cybersecurity attack detection to in-vehicle networks, with more precise identification and attack attribution, and potential response will require major actions, needed to ensure safe and secure operation.
- New explainable concepts and training of AI for CCAM applications. There is a firm need for explainable, trustworthy [4]85 AI for CCAM applications and scalable solutions, including a clear vision on the capabilities and limitations of the AI systems and AI blocks management. Concepts, techniques and models of AI for CCAM need to be developed and trained, with a first application in safety critical domains. Sufficient breadth and suitability of training data, solutions for data documentation (e.g. verifiability of data used for training), robustness and accuracy need to be incorporated. New AI approaches are to be further developed, reducing the data requirements. The new AI approaches include e.g. federated learning approaches for CCAM, edge AI and edge/cloud communication. Major improvements are to be expected in addressing user acceptance and in increasing societal impact of AI based CCAM solutions, as well as market uptake. Joint learning approaches in using AI for CCAM, but also the development and inclusion of notions of fairness in AI based tools is needed. There is a further need for approaches dealing with unknown sets of non-acquired data, and how these influence correlations, training and further implementation. Validation and harmonisation of digital tools including advanced AI technologies as EdgeAI is to be developed. This will further enhance efficient and cost-effective smart distributed and semantically aware processing tools and a flexible software infrastructure allowing distributed processing (edge/cloud) for data discovery, collection and processing alongside the product lifecycle.
- AI for situational awareness. In contributing to the essential ambitions of Vision zero, AI based CCAM and intelligent collision avoidance systems should aim to reduce the number of accidents. In this sense, AI can help by using the data provided by all road users (including vulnerable) to predict trajectories and estimate the probability of collision, and to establish on board and collective situational awareness. For meaningful (human) control of autonomous systems, advanced AI approaches –including hybrid AI- will be needed: combining system and domain knowledge, learning from data with knowledge-based AI techniques in order to improve the overall system performance, including, when needed, control handovers and handbacks. For increasing levels of automation, coordinated and comprehensive use of AI for situational awareness is essential to estimate and predict the system state, state of the human occupant or driver, and traffic state; the three parts that together determine the safety of a situation. In advancing CCAM, AI will be used to make the next steps, from situational awareness to decision-making and actuation. To limit the latency and the energy consumption of AI models, edge computing (EC) and edge intelligence (EI) will play an important role. Moreover, EC and EI allow to mitigate privacy constraints, by avoiding sharing data in the network.
- System architecture for data sharing. A harmonised approach for data sharing and data storage will be essential to allow for seamless, continuous operation by many actors (both from the vehicle side and the infrastructure) at the same time. This approach will incorporate items like data format, a common ontology for defining the terms, quality, speed, decisions on raw data vs data selections. It can help to build harmonised tools for processing data, combining inputs from multiple sources.
[4] Explainable and trustworthy AI: herewith, we mean systems which act in a for a human predictable and safe manner, which act according to clear and pre- defined ethical goal functions. The actions and decisions do follow an explainable logic, which is in line with or very close to the human decision making. As such, its actions are perceived
safe, logic and comfortable; trustworthy. It will deliver technical solutions for CCAM implementation which can be easily accepted and boost its uptake in daily use, easing potential inclusivity hurdles and maximising societal benefits of such AI-based CCAM solutions
Cluster 5 Expected Outcomes
- Safe and secure operation of CCAM vehicles and mobility systems and services, enhancing trust and end user adoption of CCAM solutions.
- Cybersecurity requirements including data security and access control enabling harmonised approaches and tools for data sharing.
- Improved understanding of the new, emerging and specific CCAM related cyber security and resilience challenges, by using the contextual definition, including sector specific security features.
- Inclusion of cybersecurity and resilience as an integral part into the development process of CCAM solutions by OEMs, Tiers, telecom providers and service providers, with common aims and objectives, frameworks/architectures and designs.
- Cybersecure data sharing approaches from pilot applications towards CCAM on a harmonised larger scale.
- Efficient and cost-effective processing tools and a flexible software infrastructure allowing distributed processing of data, as well as collection and processing alongside the product lifecycle.
- A joint and harmonised approach for data sharing and data storage, allowing for seamless and continuous operation of CCAM systems and services.
- Concepts, techniques and models based on Artificial Intelligence (AI) used for situational awareness, prediction, decision making and triggering of actions for time critical and safety relevant CCAM applications as well as for cyber threat detection and mitigation.
- A clear understanding of the capabilities, limitations and potential conflicts of AI based systems for CCAM- for the entire CCAM stakeholder community including the end users, and strategies for joint learning approaches using AI, speeding up uptake and reducing development times.
- Increased user acceptance and societal benefit, from an early stage, based on explainable, trustworthy and human-centric AI. Interactions with AI using vehicles should be understandable, human-like and reflect human psychological capabilities, including notions of fairness.
- Application of advanced AI technologies for CCAM facilitates e.g. the systems’ approach and systems’ optimisation, striving for climate friendly applications.
- Accelerated AI development and training for CCAM enabled by a relevant set of real and synthetic traffic events and scenarios.
- AI based CCAM solutions will evolve from reactive and/or adaptive system support into predictive system state awareness (including driver state and user diversity), decision-making and actuation, enhancing road safety especially in near-critical situations.
Cluster 5 Timeline (as of Feb 2024)
The Cluster 5 actions all follow a logic of a step-wise approach: moving from development on a component level (mostly in RIAs) to integration into system approaches and adjusting to needs of specific Use Cases or user groups (in IAs) ultimately to application and implementation. The first two R&I actions within Cluster 5 were planned to have an early start, with the earliest planned implementation. The third and fourth R&I actions are planned to start in the second and third year, as these actions will advance based on inputs and needs from Cluster 2, 3 and 4, with a close link to Cluster 6 for especially the R&I action on Explainable AI. The last R&I action will have its start pending on results of projects within Cluster 7 on test data sharing. Innovation Actions will follow and advance technical maturity and progress the state of the art after the first phase, delivering mature results ready for testing/implementation. The following imageaims at making this progression process transparent. As the Actions in Cluster 5 concern fairly new technologies in the application domain, the sequence of RIAs, IAs and implementation is expected to run throughout the running time of the Partnership. Based on inputs and needs from Clusters 1, 2, 3, 4 and 6, detailed (R)IA needs and implementation paths can be defined. In the same way, new key enabling technologies can arise from other sectors, for inclusion and implementation here.

Feedback form
Have feedback on this section??? Let us know!
Feedback
Please add your feedback in the field below.
Your feedback has been sent!
Thank you for your input.
An error occured...
Please try again later.